Here are four players who were projected by Steamer to steal at least 15 bags and are outperforming their wOBA projection:
Early Rabbit Returns
*Steamer Projections
Now, before you get all “snarky-comment” on me, Badoo and Thompson each only have 15 PAs and will be omitted from this analysis due to such a small sample. Only Myles Straw (46 PA) is a qualified batter, but Mateo (33 PA) is close. Yes, it’s early but just try to go into this with an open mind. For if you drafted, Myles Straw for example, you are probably pretty proud of yourself, sitting upon your thrown enjoying the grapes that are being hand fed to you while also being fanned to cool off from such a hot start. But, what can we expect from these surprises moving forward? If these are real gains, then we should expect even more stolen bases.
Myles Straw, CLE: BABIP is way up, but so is his BB%
Just look at the differences in his projected and actual batting average and wOBA. Over time, Straw will get closer and closer to that projected number. The real question is not, “Will this last?”, as much as it is, “Will he end up north or south of his projected numbers?” So far this year, he’s had a number of ground ball singles to the pull side. Here’s an example:
Straw is obviously very, very fast. But if Volpe is playing a little closer in and the third baseman doesn’t make an attempt on the ball, maybe it’s an out. It’s hard to say just how difficult of a play that was to make, but it doesn’t seem difficult to predict it doesn’t happen over and over again. It’s part of the reason Straw’s BABIP sits at .414 (current 2023 MLB average: .300). It’s completely unsustainable. Here’s a look at his spray chart and you can see a few ground ball singles to the pull side helping to inflate his BABIP:
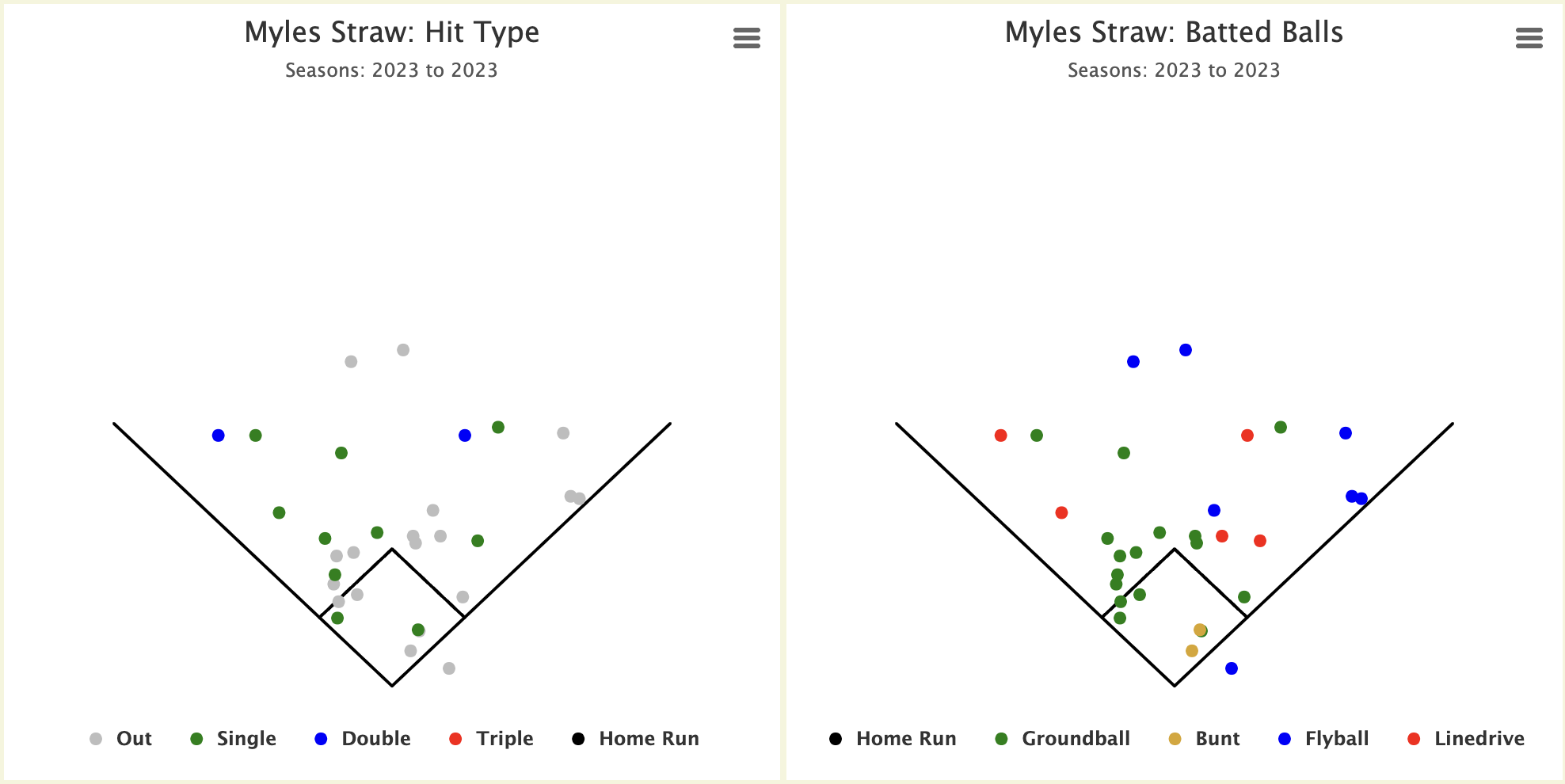
Click to enlarge
Straw is destined to regress, but how far will he regress? In order to answer that, we have to see if there has been any significant change in his approach that might suggest he has made a change. Let’s look at his O-Swing% to see if maybe he’s better at identifying bad pitches:
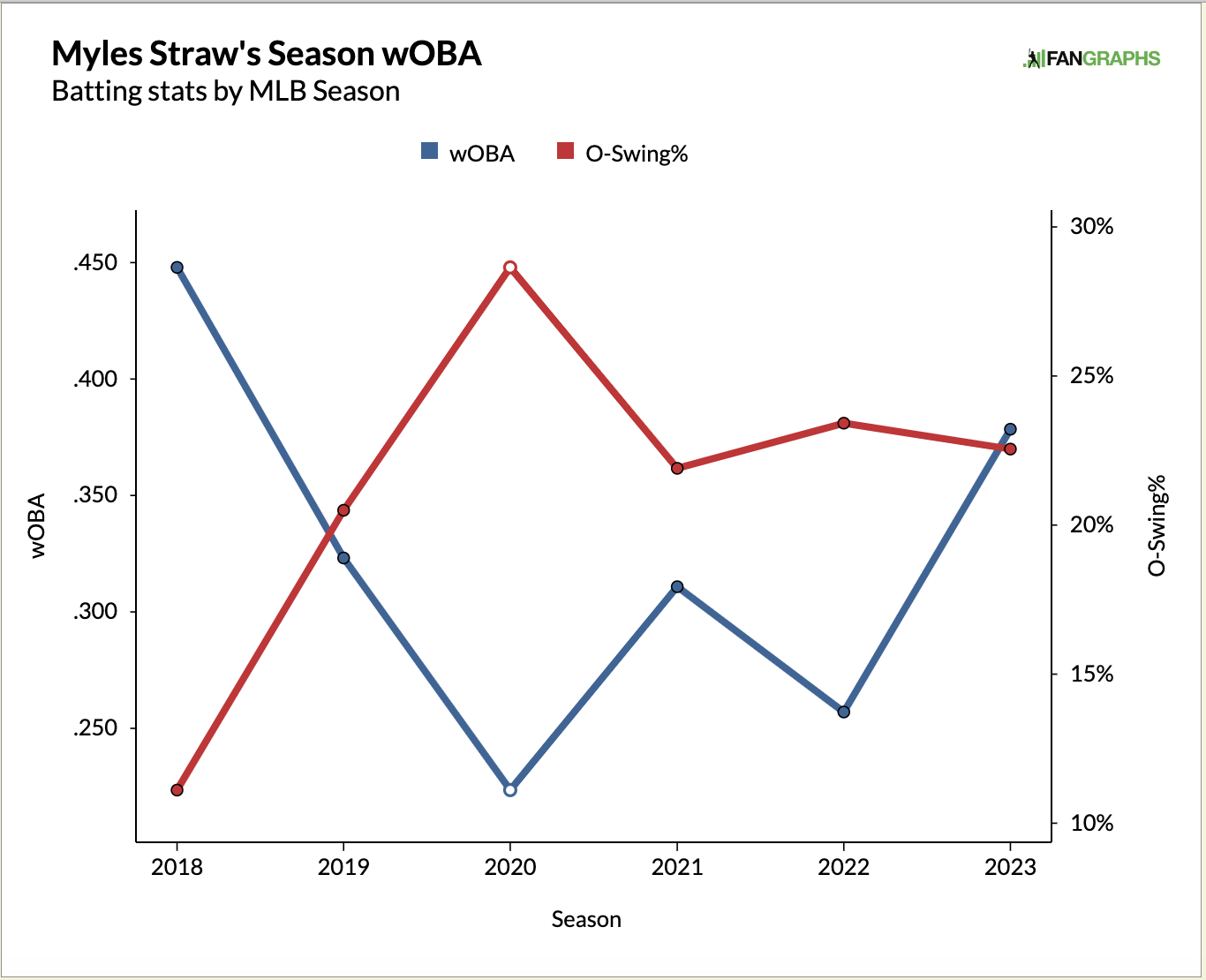
No change there. How about his approach in different counts?
Myles Straw Count Approach: 2023 vs. Career
Through Count |
2023 wOBA |
Career wOBA |
Diff |
3 – 0 |
0.706 |
0.506 |
0.200 |
3 – 1 |
0.505 |
0.459 |
0.046 |
3 – 2 |
0.502 |
0.357 |
0.145 |
2 – 0 |
0.531 |
0.412 |
0.119 |
1 – 0 |
0.453 |
0.334 |
0.119 |
2 – 1 |
0.548 |
0.354 |
0.194 |
1 – 1 |
0.545 |
0.320 |
0.225 |
0 – 1 |
0.316 |
0.261 |
0.055 |
2 – 2 |
0.445 |
0.272 |
0.173 |
1 – 2 |
0.398 |
0.218 |
0.180 |
0 – 2 |
0.178 |
0.167 |
0.011 |
*Through 50 PA in 2023
There’s some suggestion here that he has improved in 3-0 and 1-1 counts, but the sample is simply too small to make much of a conclusion from. But, if you look at his BB% towards the end of last season, he was trending in the right direction and appears to have picked up right where he left off. He’s done that before, just look at what he did in the second half of the 2021 season when he reached a peak 22.7% walk rate!
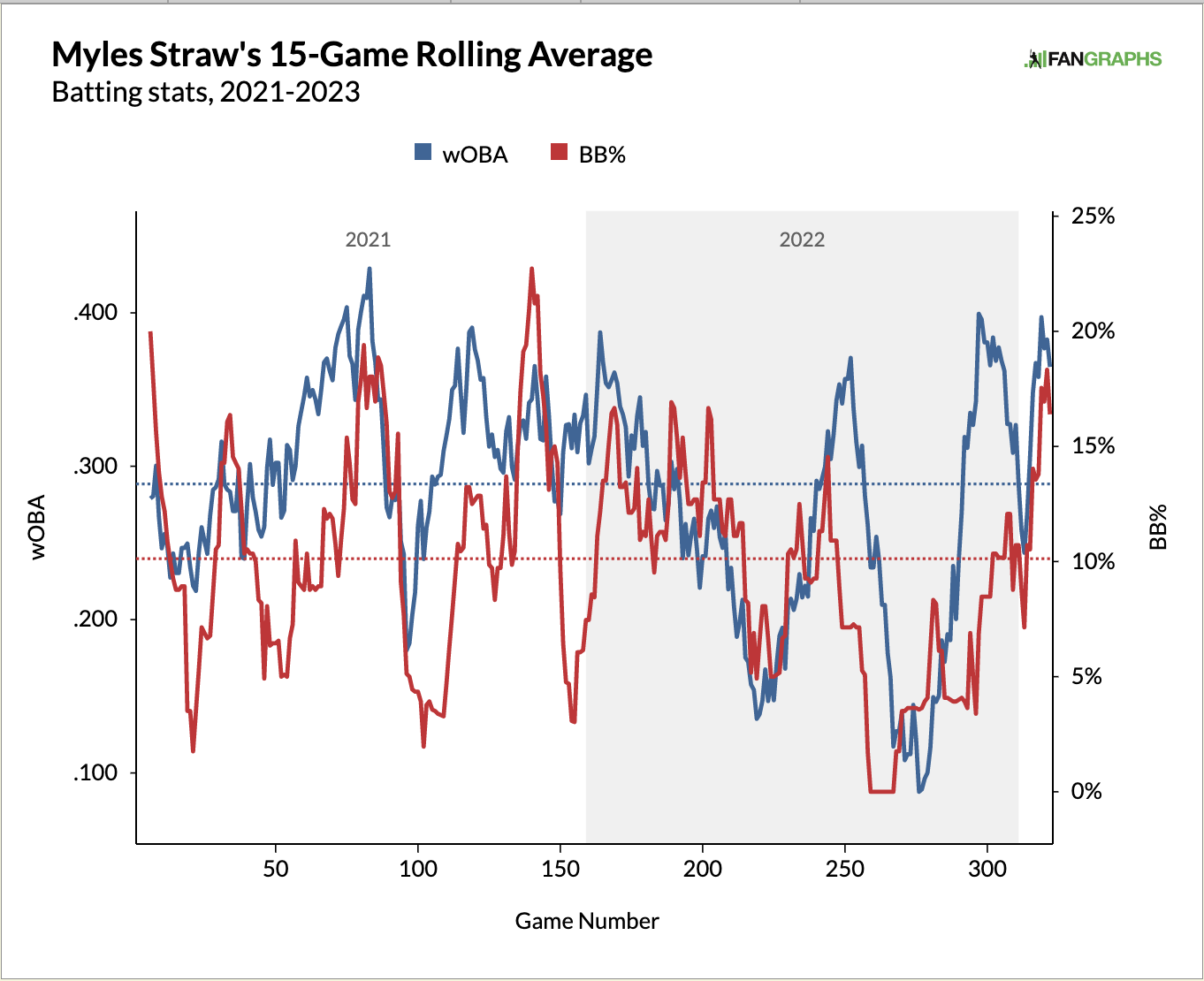
The early returns on Straw have been terrific and if you are rostering him, put him in your lineup until the well runs dry. He is an excellent base-stealer with a career 88% stolen base success rate. For context, Trea Turner has a career 85% stolen base success rate, though Turner has attempted significantly more 2B robberies. Only time will tell if his OBP (.449 2023, .326 Career) gains in the form of BABIP, wOBA, and BB% are more luck than skill.
—
Jorge Mateo, BAL: wOBA is up and plate-discipline trending in the right direction.
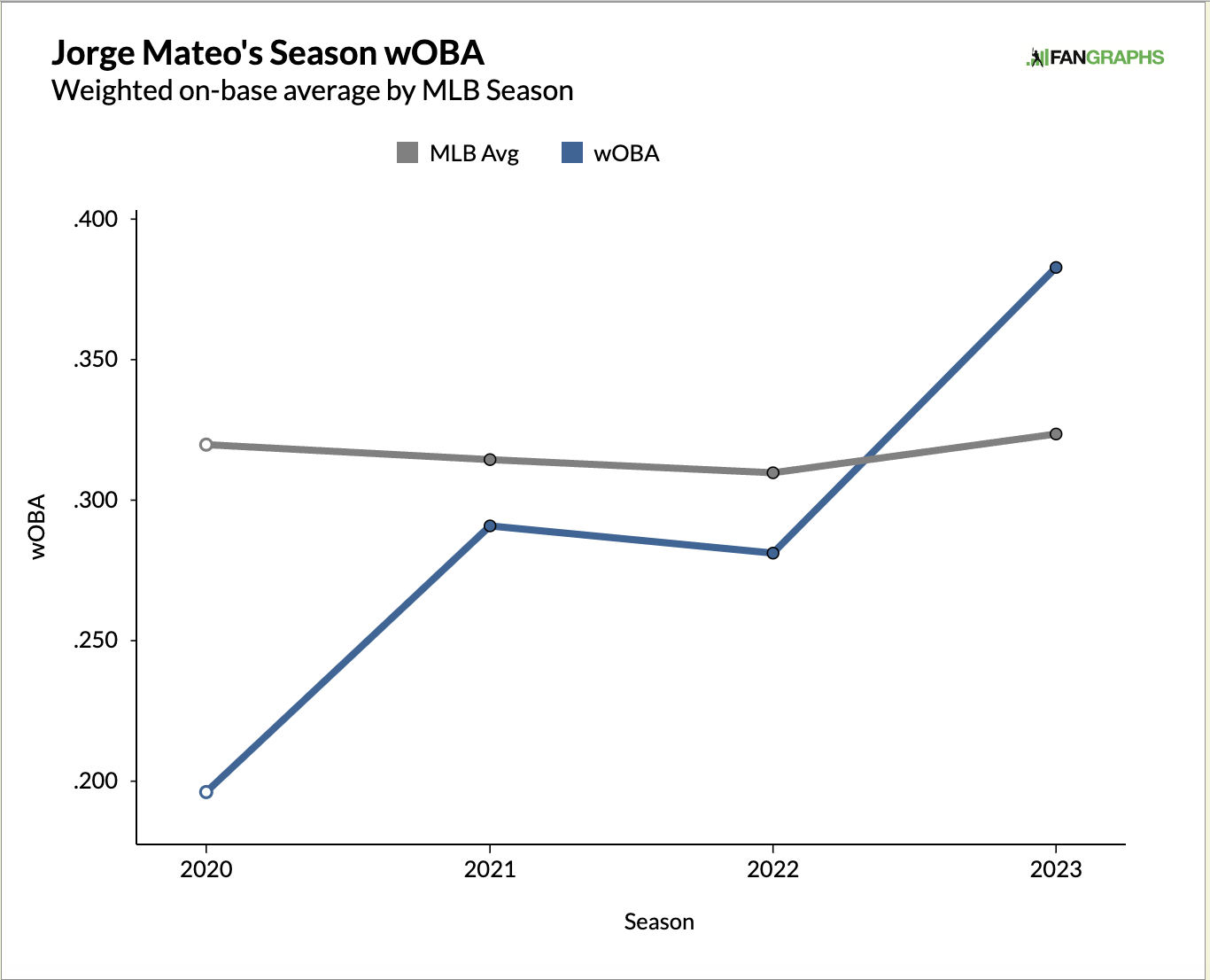
I went to my first Grapefruit League game this spring and was impressed with how good Mateo’s batted balls were looking. He just kept smashing the ball, but right at a defender. He’s always had issues with plate discipline but at the end of last season, he started to bring his rolling averages down on swings and swings outside of the zone:
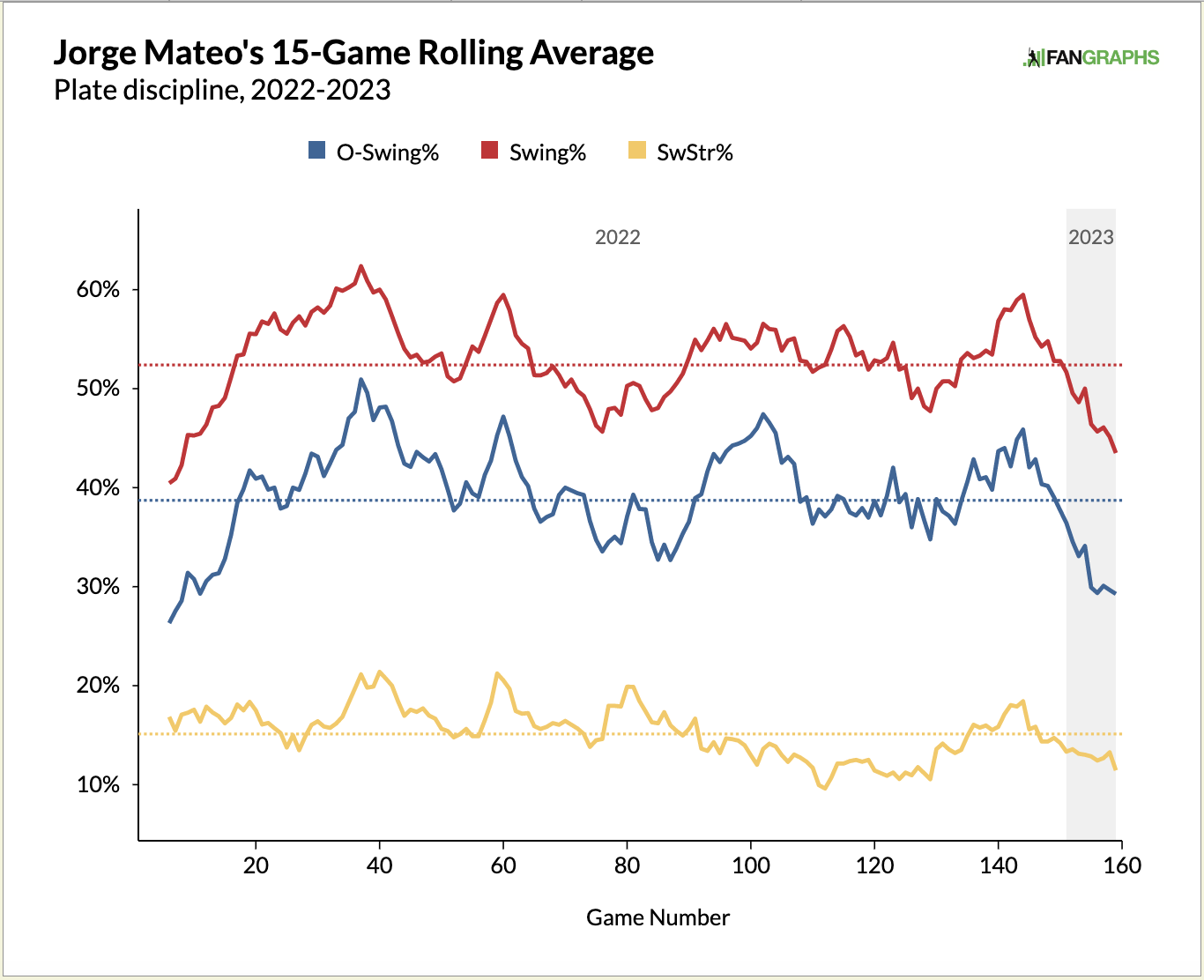
If he can work on his approach, specifically when he’s behind in the count, he could see OBP gains that would directly impact his stolen base accumulation. He is at his best when he’s ahead in the count, like in 3-1 and 2-1 counts, but he could be even better when ahead in the count. There’s no reason he shouldn’t be at or above league average in 3-0 counts. There is no reason, at all, that Mateo should be given the green light in 3-0 counts. Even if he takes and gets to a 3-1 count, he will be at his very best. So far this season, Mateo has faced four separate 3-0 counts and has taken a called strike on the next pitch in each plate appearance. That’s good. Those four plate appearances ended with a walk, a pop out, a strike out, and a hit by a pitch.
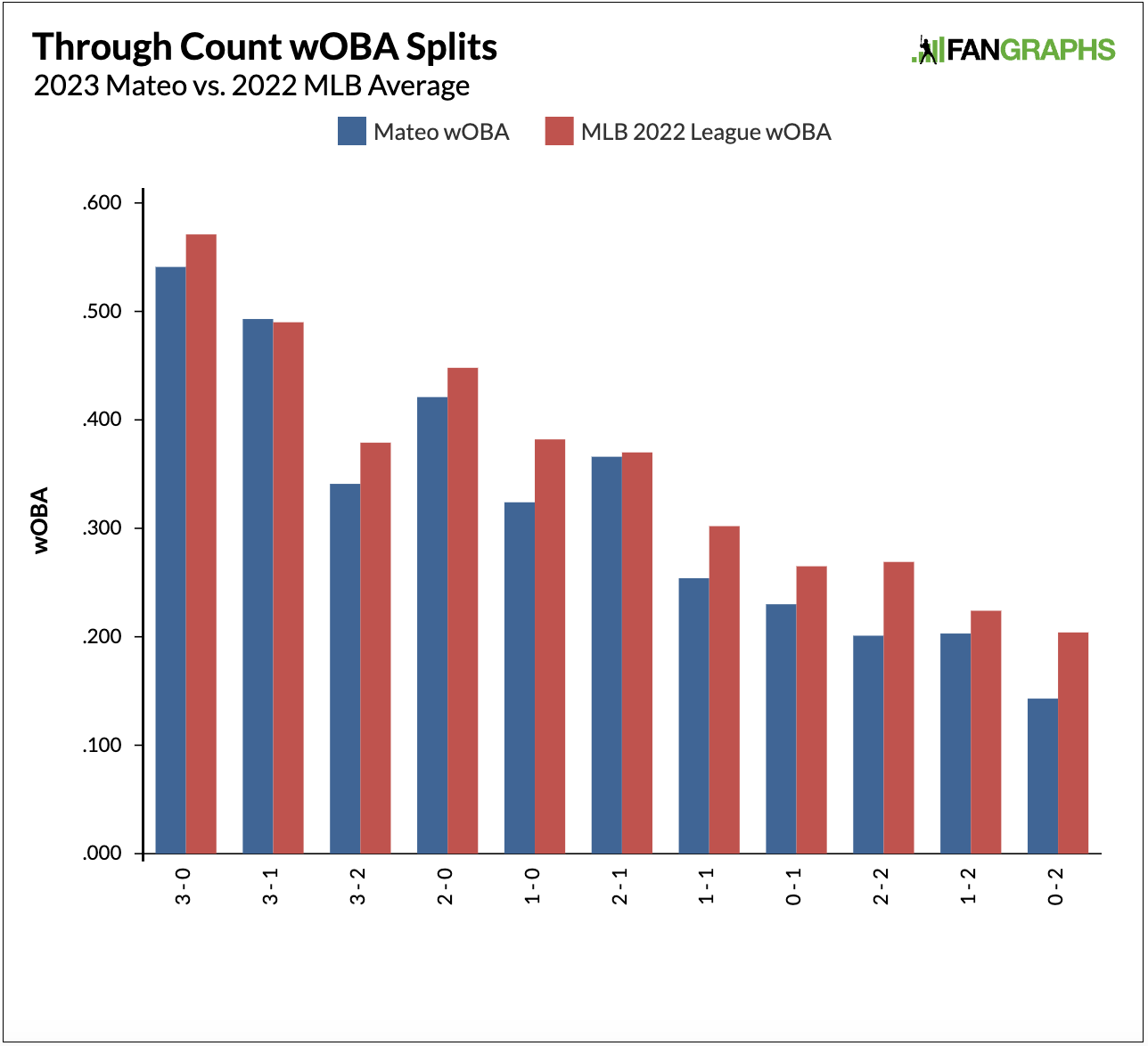
While Mateo’s plate discipline metrics are trending in the right direction, he is outperforming his expected stats, which is the opposite of what I observed in Sarasota:
AVG:.286 xAVG:.231
–
SLG:.500 xSLG:.426
–
wOBA:.383 xwOBA.324
Even still, his .324 xwOBA is just below the current league average (.328) and that’s a step in the right direction as his career-best came in 2021 when he put up a .287 xwOBA. He’s barreled the ball twice already, but that doesn’t come close to league leaders Matt Chapman and Bryan Reynolds, who each have 12 on the year. Let’s take a look at Mateo’s barreled balls:
–
Barreling balls for home runs is good fun, Mateo just needs to do it more consistently. At the end of last season, he was putting the ball on the ground less often and hitting it harder more often, and while those trends seemed like they might continue in 2023, he’ll need to increase his launch angle more consistently to make an offensive impact:
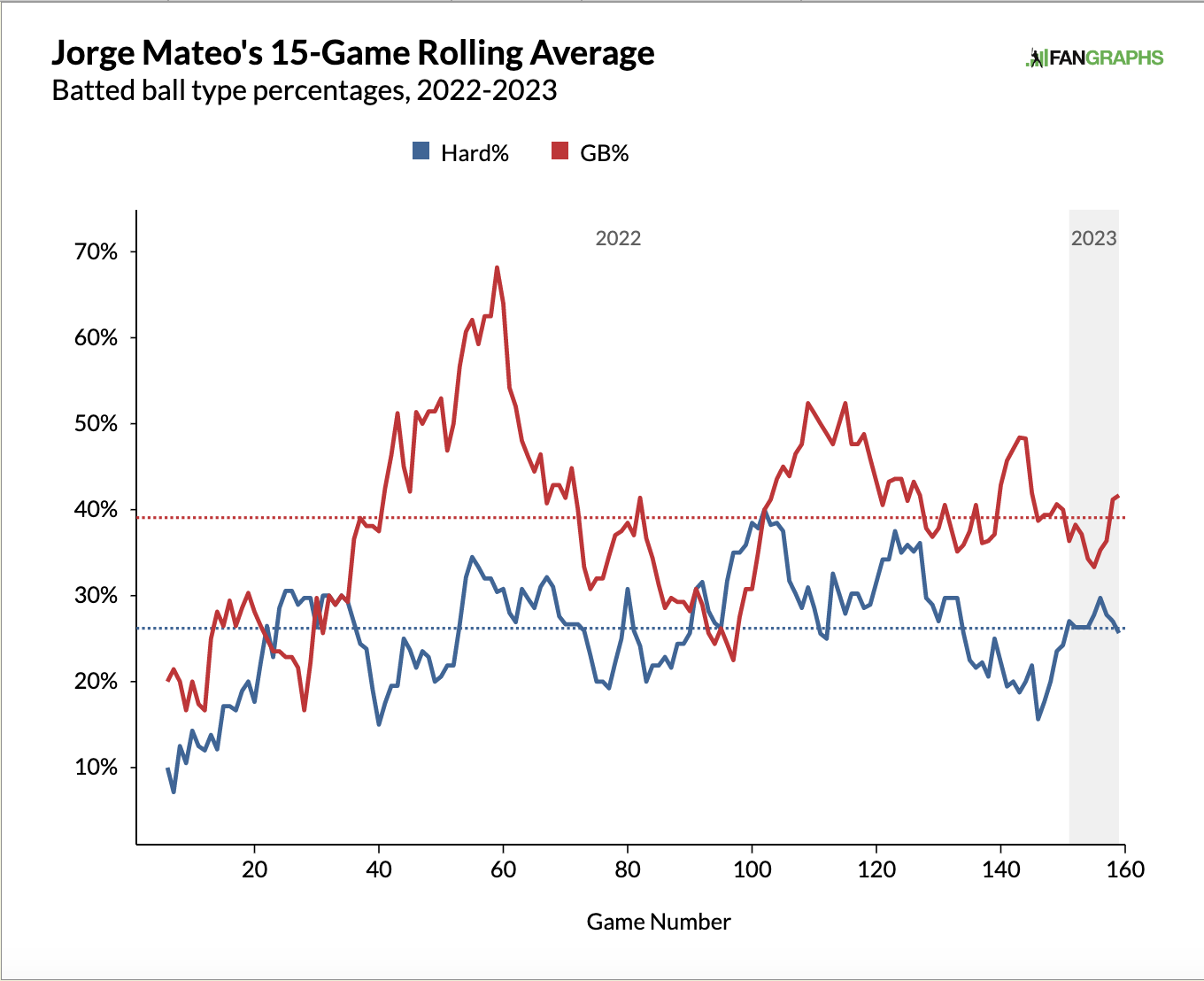
One thing is clear, base-stealers are stealing bases at rates that suggest the projections could be way off the mark by the end of the season. Now is the time to find base-stealers who have made some kind of approach or skills change that get’s them on base more often. Each one of Straw, Mateo, Badoo and Thompson should be added if they are available. If the sample size gets larger and the gains smaller, you can always drop them.
*Stats in the opening table were created on Wednesday, April 12th.