2024 Projection Review: Batter Roto Stats
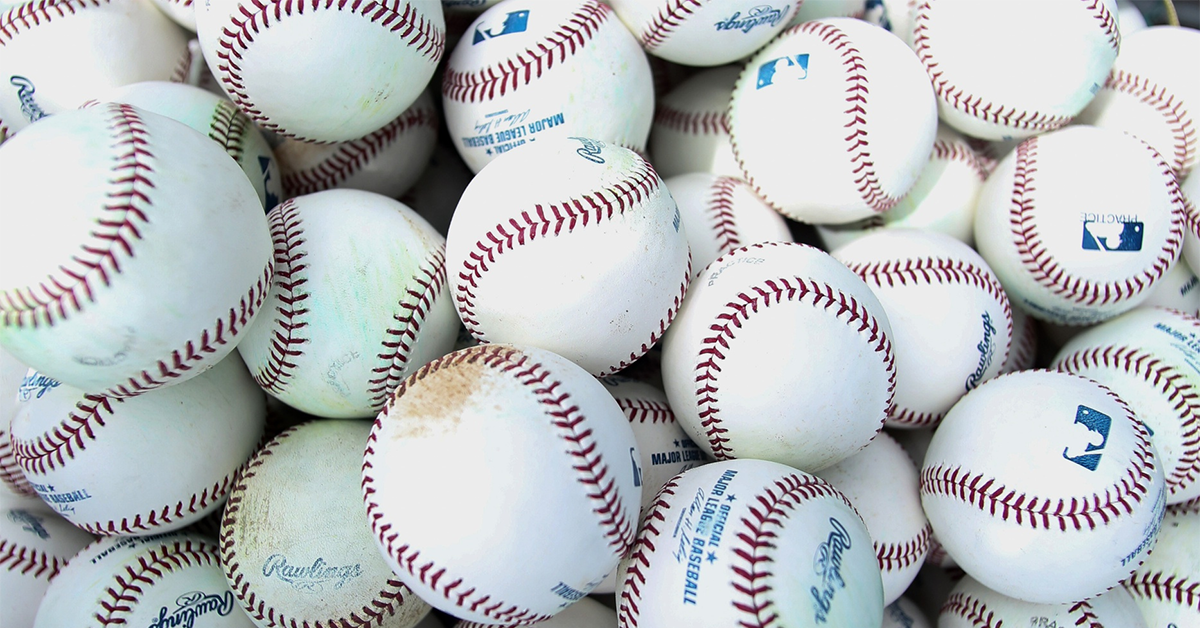
It’s time to dive into more hitter projection comparisons after examining playing time a while back. In this article, I’ll show which projections are best in the standard roto categories (R, RBI, HR, SB, and AVG) and at the end, look at ways playing time projections could be improved.
For all the background information on the test I used (RMSE) and data sample reference the first article. These tests take forever to run and at some point, I kept getting the same answers (smartly aggregating the projections), so I stopped running any new ones for hitters. Here are the results for the tests I ran.
Test 1: Batting Average
The only hitter rate stat, batting average, begins the analysis.
Projection | RMSE |
---|---|
Average | 0.0388 |
Paywall #1 | 0.0395 |
THE BAT X | 0.0406 |
4 Free Projs | 0.0406 |
ZiPS | 0.0407 |
Depth Charts (FG) | 0.0409 |
ATC | 0.0409 |
DraftBuddy | 0.0409 |
Zheile (FantasyPros) | 0.0409 |
Median | 0.0410 |
Paywall #7 | 0.0410 |
Paywall #4 | 0.0410 |
Paywall #8 | 0.0413 |
Steamer (FG) | 0.0413 |
Razzball | 0.0414 |
Davenport | 0.0415 |
Paywall #2 | 0.0415 |
Marcels (BRef) | 0.0420 |
Paywall #3 | 0.0422 |
Fantrax | 0.0425 |
Paywall #6 | 0.0428 |
Razzball (Grey) | 0.0439 |
While some individual projections are near the top, the averages and aggregators stay strong.
Test 2: Home Runs
Here is how the projections performed when looking at the raw number of home runs.
Source | HR Raw |
---|---|
Paywall #7 | 11.9 |
Marcels (BRef) | 12.0 |
Davenport | 12.5 |
Average | 12.7 |
4 Free Projs | 12.9 |
Paywall #1 | 13.0 |
ZiPS | 13.1 |
Razzball | 13.3 |
THE BAT X | 13.3 |
Median | 13.4 |
ATC | 13.5 |
Zheile (FantasyPros) | 13.6 |
DraftBuddy | 13.7 |
Paywall #6 | 13.9 |
Depth Charts (FG) | 14.0 |
Paywall #4 | 14.1 |
Paywall #3 | 14.1 |
Steamer (FG) | 14.1 |
Paywall #8 | 14.3 |
Fantrax | 14.3 |
Razzball (Grey) | 14.4 |
Paywall #2 | 14.6 |
With Paywall #7 and Marcels crushing the playing time estimates, it’s no surprise they are at the top. And here are the home projections prorated per plate appearance.
Source | HR/PA |
---|---|
Paywall #7 | 0.0184 |
Average | 0.0186 |
Davenport | 0.0188 |
Paywall #1 | 0.0191 |
4 Free Projs | 0.0196 |
Marcels (BRef) | 0.0198 |
Median | 0.0199 |
Depth Charts (FG) | 0.0199 |
ZiPS | 0.0200 |
Steamer (FG) | 0.0200 |
ATC | 0.0202 |
THE BAT X | 0.0202 |
Razzball | 0.0202 |
DraftBuddy | 0.0202 |
Paywall #3 | 0.0208 |
Paywall #2 | 0.0209 |
Paywall #8 | 0.0211 |
Paywall #4 | 0.0213 |
The big surprise was that Marcels remained near the top even when the home runs were turned into a rate stat. The aggregators held up but weren’t as strong as previous tests.
Test 3: Stolen Bases
Here are the stolen bases projections ranked by the raw number
Projection | SB |
---|---|
Average | 8.50 |
Razzball | 8.61 |
4 Free Projs | 8.73 |
Davenport | 8.80 |
Paywall #7 | 8.87 |
Paywall #8 | 8.93 |
Median | 8.95 |
Zheile (FantasyPros) | 9.00 |
ZiPS | 9.05 |
Steamer (FG) | 9.05 |
THE BAT X | 9.08 |
Marcels (BRef) | 9.09 |
ATC | 9.09 |
Depth Charts (FG) | 9.10 |
DraftBuddy | 9.11 |
Paywall #6 | 9.12 |
Fantrax | 9.33 |
Paywall #2 | 9.40 |
Paywall #1 | 9.42 |
Paywall #3 | 9.56 |
Razzball (Grey) | 9.73 |
Paywall #4 | 9.81 |
A new order of projections on this raw stat … well besides the averages performing near the top. Here they are as a rate stat.
Projection | SB/PA |
---|---|
4 Free Projs | 0.0132 |
Median | 0.0132 |
Average | 0.0132 |
Depth Charts (FG) | 0.0132 |
ATC | 0.0133 |
Razzball | 0.0133 |
DraftBuddy | 0.0133 |
ZiPS | 0.0133 |
Steamer (FG) | 0.0133 |
THE BAT X | 0.0134 |
Paywall #2 | 0.0134 |
Marcels (BRef) | 0.0134 |
Davenport | 0.0136 |
Paywall #1 | 0.0139 |
Paywall #3 | 0.0139 |
Paywall #7 | 0.0139 |
Paywall #4 | 0.0141 |
Paywall #8 | 0.0142 |
That’s some domination by the aggregators by taking the top five spots.
Test 4 Runs plus RBI (R+RBI)
I combined the two because the results were consistent (aggregators kicking ass) and I just wanted to see if any of the results stood out like with stolen bases.
Source | R+RBI |
---|---|
Marcels (BRef) | 57.7 |
Paywall #7 | 57.7 |
Average | 61.9 |
Davenport | 62.6 |
4 Free Projs | 63.8 |
THE BAT X | 64.0 |
Razzball | 64.8 |
ATC | 64.8 |
Paywall #4 | 65.5 |
Median | 65.8 |
DraftBuddy | 66.2 |
ZiPS | 66.5 |
Zheile (FantasyPros) | 66.8 |
Paywall #6 | 67.4 |
Paywall #1 | 68.0 |
Paywall #3 | 68.0 |
Fantrax | 68.3 |
Paywall #8 | 68.7 |
Steamer (FG) | 69.2 |
Razzball (Grey) | 69.5 |
Depth Charts (FG) | 70.7 |
Paywall #2 | 71.5 |
Nothing changed. Correctly guessing playing time allows a projection to dominate these rankings. It’s time to move on.
Conclusions on Hitter Projections
The answer is simple, get an aggregation of projection. ATC and Zeile already do the combination. Or a person could use Tanner Bell’s projection aggregator to personally control the inputs and weighting.
Additionally, if combining projections, I would not pay up for any with Razzball, ZiPS, THE BAT X, and Davenport all performing great.
Test 5: Where Projections Miss
Note: I cut and diced the available information in what seemed a 100 different ways. The following are the two best examples I found for why projections miss. I’m sure there are better ways to improve projection playing but I haven’t havent figured them yet.
From some of my unpublished work, I have determined that projections miss based on age, previous playing time (proxy for health), and talent (projected OPS). I wanted to find out why Marcels performed better than the standard projections. In 2024, here are the players the 4 Big Projs projected for more playing time than Marcels.
Name | Position | 4 Free Projs – Marcel |
---|---|---|
Evan Carter | OF | 80.7 |
Oneil Cruz | SS | 78.5 |
Rhys Hoskins | 1B | 75.0 |
Christian Encarnacion-Strand | 1B | 66.5 |
Vinnie Pasquantino | 1B/DH | 63.4 |
Ceddanne Rafaela | SS/OF | 59.5 |
Parker Meadows | OF | 53.2 |
Elly De La Cruz | SS | 47.6 |
Royce Lewis | 3B | 44.7 |
Trevor Story | SS | 44.5 |
Nolan Jones | OF | 44.1 |
Sal Frelick | OF | 42.7 |
Zack Gelof | 2B | 42.6 |
Logan O’Hoppe | C | 37.4 |
Jordan Walker | OF | 35.9 |
Riley Greene | OF | 33.9 |
A table full of prospects (e.g. Carter, Cruz) or injured players (e.g. Lewis, Hoskins). This verifies some of my previous findings that players with checkered playing histories miss their playing time projections.
Dropping the playing time on hurt guys is not hard but it is tougher with guys like Elly. If he plays every day, he’s a steal.
For the next example, I grouped hitters by their Marcel playing time projection and combined plate appearances from the previous two seasons. Then I compared our 2021 to 2024 projected Steamer plate appearances to the actual number. Here are the results.
Marcels (.5 * Prev + .1 * Prev2 + 200) | |||
---|---|---|---|
PA | PA | Avg PA Diff | Avg OPS |
525 | 650 | 38.3 | 0.797 |
350 | 525 | 45.9 | 0.749 |
200 | 350 | 22.0 | 0.719 |
Previous 2 seasons PA total | |||
Min PA | Max PA | Avg PA Diff | Avg OPS |
1100 | 1500 | 36.1 | 0.797 |
700 | 1100 | 56.7 | 0.765 |
0 | 700 | 24.2 | 0.724 |
Steamer’s plate appearance projections perform great for regulars or bench bats. The players between those two are the toughest to estimate with over 500 PA in each previous season needed for a solid playing time projection.
While I focused on our Steamer projections, all of the other projection systems over project playing time compared to Marcels. They likely had the same issues. A fantasy manager might need to some way take into previous playing time while making future estimates.
For right now, I don’t know the right answer. As a group, it is a little embarrassing that a simple formula kicked everyone’s collective ass in playing time. In the previous article, I mentioned adding in a computer projection (e.g. Marcels) to temper expectations. I stand by that observation. For now, that’s all I can recommend.
Jeff, one of the authors of the fantasy baseball guide,The Process, writes for RotoGraphs, The Hardball Times, Rotowire, Baseball America, and BaseballHQ. He has been nominated for two SABR Analytics Research Award for Contemporary Analysis and won it in 2013 in tandem with Bill Petti. He has won four FSWA Awards including on for his Mining the News series. He's won Tout Wars three times, LABR twice, and got his first NFBC Main Event win in 2021. Follow him on Twitter @jeffwzimmerman.
Jeff, great piece! This (appears to) confirm something I’ve been thinking about over the last year or two. Basically, projection systems are not equally good at projecting each category. This analysis shows that the WORST projection for SB or HR had an RMSE more than twice as low as the BEST projection for AVG.
My theory that I’m play-testing this year is that I believe having equal weights for the categories (HR, SB, AVG, R+RBI) in a SGP/Z-score calculator actually leads to less accurate values overall. HR and SB should be considered more heavily when basing dollar values/analysis on projections simply because their projections are generally more reliable.
I’m not saying to ignore average or to discount it too heavily (obviously it’s super important), but the dollar value component from batting average is less reliable than the others and should not be considered of equal weight.
Do you think I’m barking up the wrong tree entirely or is there something interesting along this line of thinking?